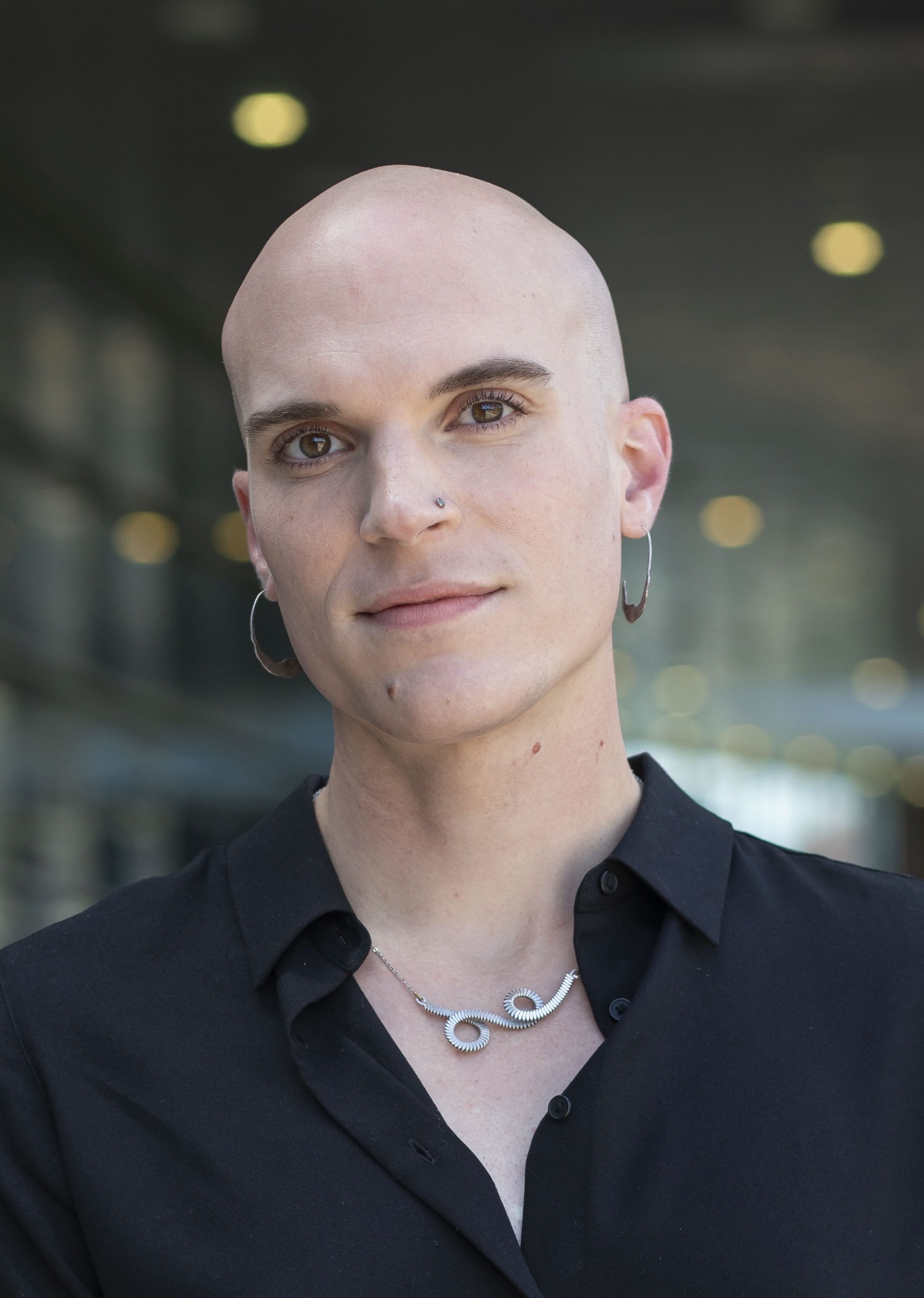
dr. dana calacci
she/her they/them
assistant professor, Penn State IST
I study how data and AI impact communities.
Right now, I'm focused on how algorithmic management is changing the reality of work, how data stewardship and participatory design can help create alternate working futures, and on the data rights of platform workers.
🔥 recruiting students 🔥
I'm looking for PhD students to join me in building my lab at Penn State. Interested? Send me an email with 'building working futures' in the subject and tell me why you want to join. Please review my recent papers before cold e-mailing me.
Research Areas
Data Rights as Labor Rights
While workplaces are increasingly quantified and surveilled, normative and legal notions of data privacy conflict with property, trade secret, and IP law in ways that limit worker data access.
Data Tools For Workers
In a working reality that is increasingly algorithmically managed, workers, researchers, and advocates need tools to manage data and develop alternate algorithmic futures.
Corporate Surveillance of the Commons
Amazon Ring is the US's fastest-growing private surveillance network. Where is it, how do people use it, and what should we do about it?
Urban Segregation and Mobility Behavior
Economic and racial Segregation are structural and emergent phenomena, created by institutional processes and individual behavior alike. How can we measure and change the segregation in cities across the globe?
News
- Nature Scientific Data2024Open e-commerce 1.0, five years of crowdsourced US Amazon purchase histories with user demographics
Alex Berke, D Calacci, Robert Mahari, Takahiro Yabe, Kent Larson, Sandy Pentland
The result of our crowdsourcing experiment, this dataset details the purchase histories of over 5,000 users over 5 years. We show a few ways the data can be used and validate its' representativeness.
- CHI Workshop on LLMs and Qualitative Research2024QuaLLM: An LLM-based Framework to Extract Quantitative Insights from Online Forums
Varun Rao, Eesha Agarwal, Samantha Dalal, D Calacci, Andrés Monroy-Hernandez
This work experiments with using LLMs to analyze and extract specific insights from large unstructured text corpora (such as all the posts on the rideshare subreddits).
- (under submission)2024Insights from an experiment crowdsourcing data from thousands of US Amazon users: The importance of transparency, money, and data use
Alex Berke, Robert Mahari, Alex (Sandy) Pentland, Kent Larson
We investigate how different incentive structures impact people's willingness to contribute their Amazon purchase history data to an academic research project. One big takeaway: data transparency matters for crowdsourced data participants.
- Princeton Digital Witness Lab2023Princeton CITP Digital Investigators Conference
D Calacci
I was invited to speak at this invite-only event that convened investigative journalists, non-profit researchers, academics, and activists to discuss the promises and challenges of doing data-driven investigatory work in tech accountability.
- European Labor Law Journal2023From access to understanding: Collective data governance for workers
D Calacci & Jake Stein
How does current data protection law work for workers? In this invited article for a special issue of the European Labor Law Journal, my colleague Jake Stein and I argue that worker co-determination should be seriously considered as a way to regulate AI and data use in the workplace.
- ACM Interactions2023Building Dreams Beyond Labor: Worker Autonomy in the Age of AI
D Calacci
Contribution to ACM Interactions' Tech + Labor Forum on automation and worker agency. I argue that workers—and researcher+advocate allies—can & should collectively help direct automation at work.
- MozFest 20232023MozFest Panel: Navigating the open-source algorithm audit tooling landscape
Dan Calacci, Deb Raji, Abeba Birhane, Brandi Geurkink, Becca Ricks, Claire Pershan, Mehan Jayasuriya, Victor Ojewale, Marc Faddoul
I participated in an invited panel discussion on the state of algorithmic auditing tools! This was a great discussion that touched on the new Digital Services Act, community control of data and auditing technologies, and the taxonomy of audit tools.
- CSCW2022The Cop In Your Neighbor's Doorbell: Amazon Ring and the Spread of Participatory Mass Surveillance
D Calacci, Jeffrey Shen, Alex (Sandy) Pentland
We use spatial regression models, structured topic models, and an experimental survey to understand how users of Amazon's Ring Neighbors network racialize and criminalize their subjects, and document what kinds of communities nationwide use the network most.
- ACM CCS2022Privacy Limitations Of Interest-based Advertising On The Web: A Post-mortem Empirical Analysis Of Google's FLoC
Alex Berke, D Calacci
In 2020, Google introduced FLoC, a way to facilitate interest-based individual advertising without 3rd-party cookies. This paper shows that the FLoC proposal included serious privacy risks and explores FLoC's risk of leaking sensitive demographic information about it's users.
- CSCW2022Bargaining With the Black-Box: Designing and Deploying Worker-Centric Tools to Audit Algorithmic Management
D Calacci, Alex (Sandy) Pentland
This paper introduces the Shipt Calculator, a tool used in a 2020 worker-led campaign that revealed that Shipt's new black-box payment algorithm cut the pay of over 40% of studied workers.
- FTC2022Invited panelist at FTC PrivacyCon 2022
I was an invited panelist at FTC's PrivacyCon in November 2022, speaking about commercial and worker surveillance. In my talk, I argued that data regulation for workers should be more about worker power and agency than privacy rights.
- The Guardian2022Work featured in The Guardian: Porch piracy: are we overreacting to package thefts from doorsteps?
Lam Thuy Vo
Our work on Amazon Ring was featured heavily in an investigative piece published by the Guardian and Type Investigations examining the rise of new laws in the US turning package theft into a felony.
- Mozilla IRL Podcast2022Mozilla Internet Health Report 2022
Mozilla
I was featured in the 2022 Mozilla Internet Health Report. This year, it took the form of a podcast where I was featured in an episode about workers, organizers, and researchers thinking about how technology and data impact modern work.
- HOPE 20222022HOPE 2022 Invited Talk: Hacking a Path to Data-Driven Organizing
D Calacci
In July 2022, I gave an invited talk at the Hackers on Planet Earth conference outlining some existing projects related to using data for worker organizing, and discussing more generally how a hacker ethos can fit within the modern labor movement.
- NPR2022Radiolab: Gigaverse
WNYC's Radiolab
I appeared on Radiolab's August 26, 2022 episode, sharing my experience working with worker-organizers to audit Shipt's black-box pay algorithm and to discuss the condition of gig workers more generally.
- FAccT2022Keynote: How to Bargain with a Black Box: Auditing an Algorithmic Pay Change With a Community-Led Audit
Willy Solis, Vanessa Bain, D Calacci, Drew Ambrogi, Danny Spitzberg
A real-world community audit of a black-box algorithmic system, the Shipt Calculator impacted workers, organizers and researchers and demonstrates how community-led research can be part of the FAccT community.
- CHIWORK2022Organizing in the End of Employment: Information Sharing, Data Stewardship, and Digital Workerism
D Calacci
Position paper in CHIWORK '22 arguing that a new "Digital Workerism" in the CHI and CSCW communities is needed to bolster the labor movement and balance information asymmetries.
- Op-Ed2022Google Needs to Unlock Its Ad Privacy Black Box
Gizmodo
Google's FLoC was a proposal that would change the way the web fundamentally worked for millions of people. Why was studying it so inaccessible? In this Op-Ed, I argue that centralized gatekeeping of future web technologies is dangerous for the future of the web. I call for Google and other major companies to publish toolkits that let researchers study new technologies that will fundamentally change the web.
- Nature Comms.2021Mobility patterns are associated with experienced income segregation in large US cities
Esteban Moro, D Calacci, Xiaowen Dong, Alex (Sandy) Pentland
Is your local coffee shop more segregated by income than your favorite movie theater? We use a massive data set of mobile phone mobility to answer this question and model how individual segregation is related to people's tendency to explore new places and interact with those different than themselves.
- Data & Society2020Data & Society: Cop in Your Neighbor's Doorbell
D Calacci
Invited talk at Data & Society on mapping and analyzing Amazon Ring's network.
- HOPE2020One Ring to Surveil Them All: Hacking Amazon Ring to Map Neighborhood Surveillance
D Calacci
Remote presentation at HOPE (Hackers On Planet Earth) 2020 on hacking Amazon Ring's Neighbors app to reveal and measure the extent of neighborhood surveillance captured in the Ring Doorbell camera network.
- AAMAS2020Leveraging Communication Topologies Between Learning Agents in Deep Reinforcement Learning
Dhaval Adjodah, D Calacci, Abhimanyu Dubey, Anirudh Goyal, P.M. Krafft, Esteban Moro, Alex Pentland
Can network structures inspired by human social networks improve distributed reinforcement learning algorithms? This paper proves that arranging agents in different network topologies can massively improve evolutionary deep reinforcement learning algorithms.
- Op-Ed2020Location Tracking To Fight Coronavirus Is Dangerous And Possibly Pointless
Gizmodo
At the beginning of COVID, many states and universities were experimenting with using location data to track covid spread. In this op-ed I argued that location data is a dangerous technology to break out for state-level disease surveillance and is a poor technical choice for tracking airborne illness.
- Preprint2019The Tradeoff Between the Utility and Risk of Location Data and Implications for Public Good
D Calacci, Alex Berke, Kent Larson, Alex (Sandy) Pentland
Location data collected from mobile phones and aggregated in massive databases poses enormous risks to individual and collective privacy. It also poses clear utility for research, marketing, and policymaking. This paper explores and conceptually models the risks that large-scale location datasets introduce, and speculates on ways that location data can be regulated or protected while offering significant utility.